Aug 10
/
Manisha Arora & Julian Hsu
Making Data-Driven Decisions: Navigating the Challenges of Business Experiments - Part 1/4
In the business world, experiments serve as critical tools to guide strategic decision-making and product development. They help us decide which path to take when the strategy is at the fork in a road. Experimentation plays a crucial role in developing, improving, and scaling products and services. It brings data-driven recommendations to discussions such as “what price your products should be”, and “how to move more customers through your funnel”.
By conducting experiments, tech companies can gather insights about user behavior and test hypotheses of how to improve their products. This allows them to enhance their product experience, and effectively reach a larger scale.
By conducting experiments, tech companies can gather insights about user behavior and test hypotheses of how to improve their products. This allows them to enhance their product experience, and effectively reach a larger scale.
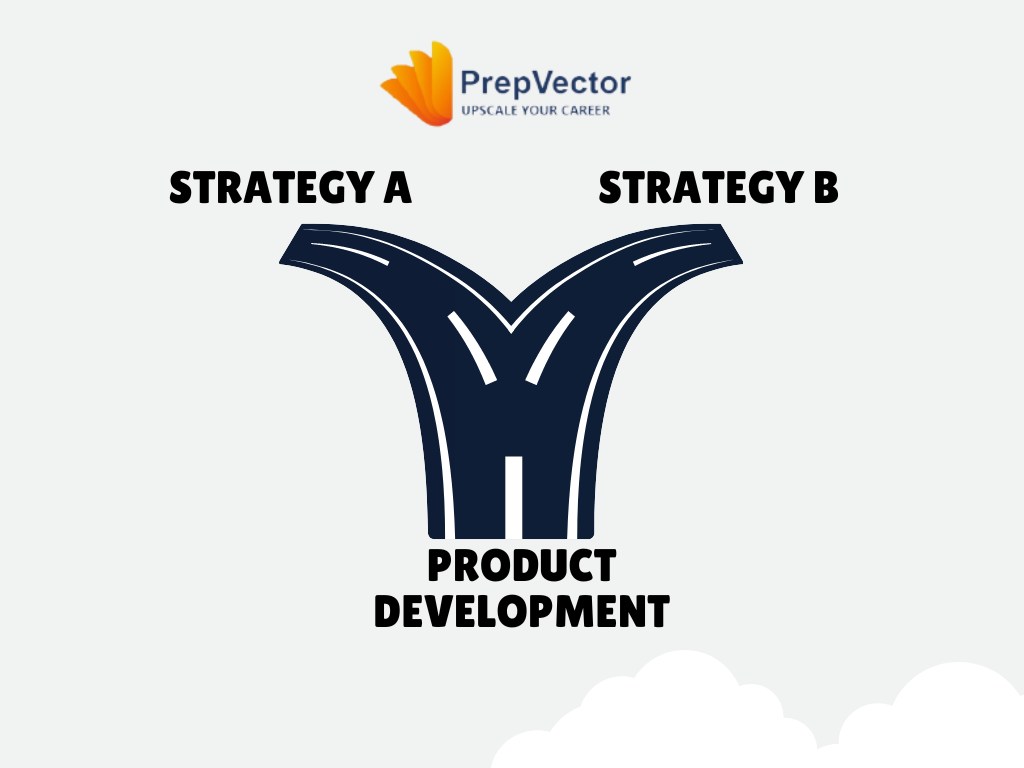
The Challenge of Effective Experimentation:
While experimentation is a valuable approach, it is not as simple as flipping a coin to compare treatment and control groups. Setting up experiments requires careful consideration of several difficult questions:
Determining Sample Size: How large should our sample size be to ensure reliable results?
Experiment Duration: How long should the experiment run to achieve a sufficient sample size and obtain confident results?
Budget and Time Constraints: Can we obtain reliable results within budget and time constraints?
These questions can initially seem daunting, leaving us wondering why we must impose limitations before even starting the experiment. After all, isn't the purpose of an experiment to explore and collect data? However, it is essential to approach experiments with a specific decision and measurable goal in mind. This mindset shift allows for a more structured approach to the problem, reducing the risk of making decisions based on random noise rather than the intended treatment.
These questions can initially seem daunting, leaving us wondering why we must impose limitations before even starting the experiment. After all, isn't the purpose of an experiment to explore and collect data? However, it is essential to approach experiments with a specific decision and measurable goal in mind. This mindset shift allows for a more structured approach to the problem, reducing the risk of making decisions based on random noise rather than the intended treatment.
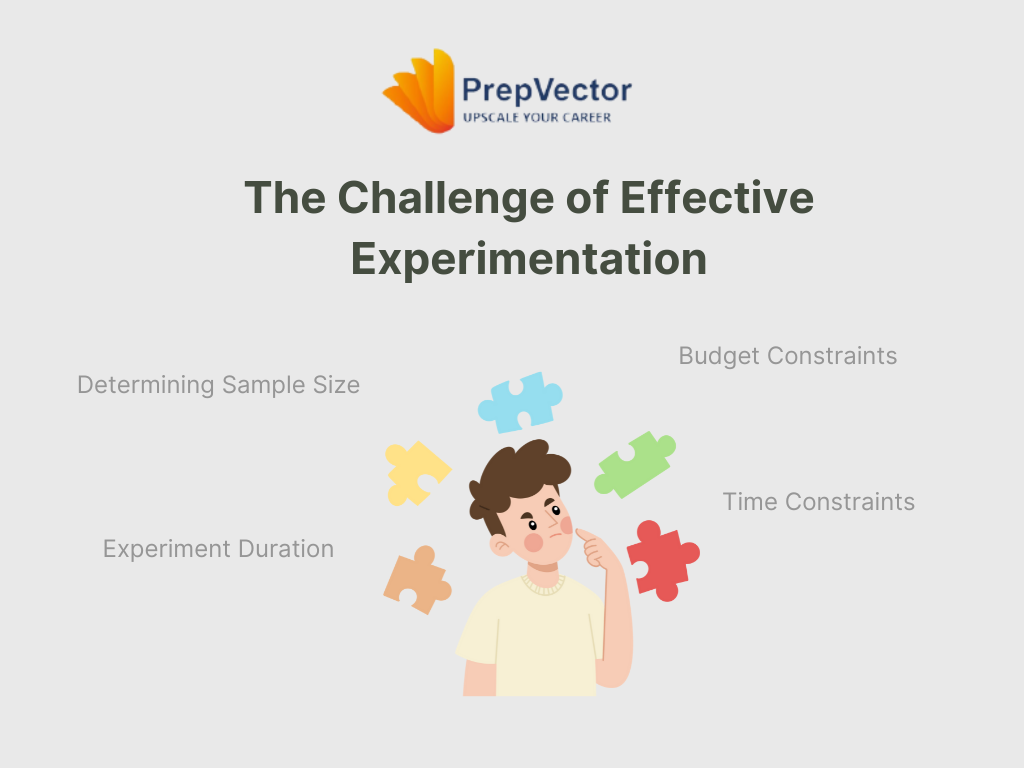
From Exploration to Decision-Making:
Instead of designing an experiment just to explore, we often instead design experiments to make a decision. That decision could be whether to launch a treatment (ie website UI design element) across all units, whether we need to refresh model parameters for an online tool (ie whether last year’s decision to have 10 search results per page maximizes profit), or whether we can launch an experiment given a set budget (ie pay respondents to go through an experiment).
When we pivot our thinking that experiments inform a particular decision and measurable goal, we can apply some more structure to our problem and make progress.
When we pivot our thinking that experiments inform a particular decision and measurable goal, we can apply some more structure to our problem and make progress.
This structure lets us navigate the risk of making recommendations that are plagued by random noise in the data rather than the treatment we wanted to test, wasting project budget on an experiment that would inevitably generate low-confidence results. When we answer the following questions, we can make the most of our experiment.
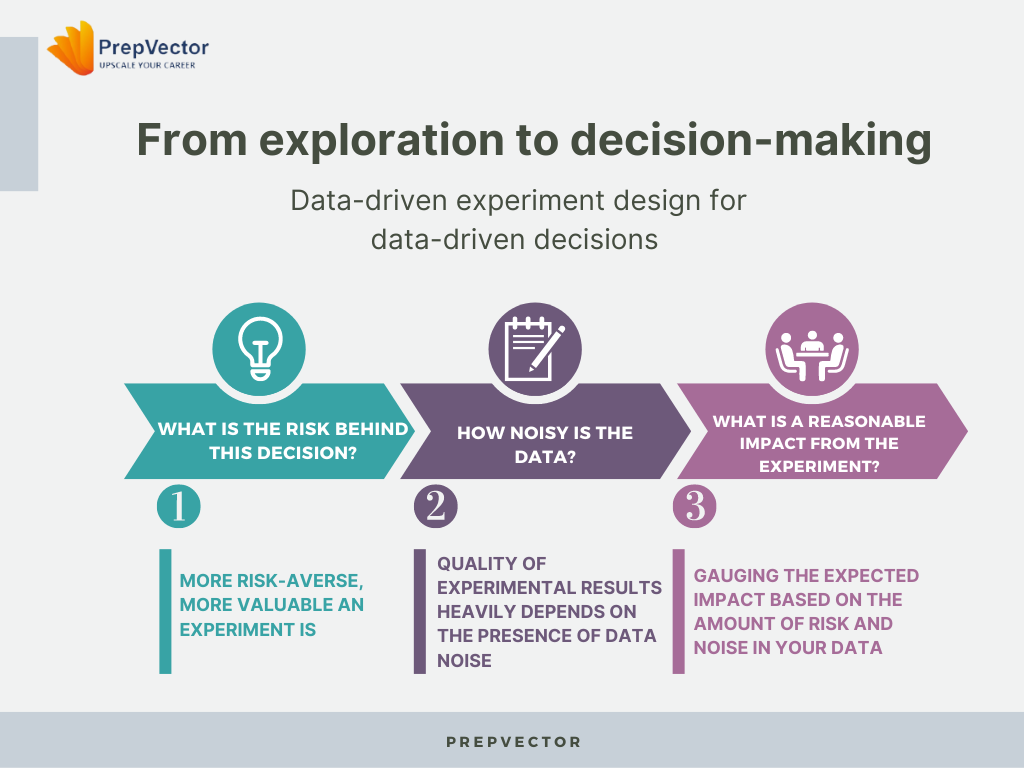
1. What is the risk behind this decision?
Your experiment may improve your product or not. Since you do not know until you try, you need to consider how much risk you are willing to take on. Depending on the stakes, we may be more willing to launch a new service even when it’s no better or even worse than the current status. In contrast, the more risk-averse we are, the more valuable an experiment is to us.
2. How noisy is the data?
The quality of experimental results heavily depends on the presence of data noise. The noisier the data is, the more likely it is that we think our experiment worked. To mitigate this challenge, we can adopt several strategies, such as increasing the sample size or using sophisticated machine learning models to reduce noise.
Additionally, extending the duration of the experiment can yield more data. However, it's crucial not to overwhelm the experiment by testing too many treatments simultaneously, as this could compromise the reliability of the results.
For example, if you have 100 customers, an experiment that splits them into two groups is more reliable than if you split them into one hundred groups.
Additionally, extending the duration of the experiment can yield more data. However, it's crucial not to overwhelm the experiment by testing too many treatments simultaneously, as this could compromise the reliability of the results.
For example, if you have 100 customers, an experiment that splits them into two groups is more reliable than if you split them into one hundred groups.
3. What is a reasonable impact from the experiment?
An integral part of experiment design involves gauging the expected impact. Based on the amount of risk and noise in your data, you can calculate the minimum detectable effect (MDE). If the MDE is greater than the impact you expect to have, then you cannot determine whether your experimental result is due to noise or the experiment itself.
Some of these questions may seem counterintuitive. If we want to have data-driven decisions, then shouldn’t we also have a data-driven experiment design?
Experiments require resources: budgets to collect data, engineering know-how and effort to scale treatments, and designer resources on how to design different treatments to name a few. Answering the above questions will help you determine what it will take to design and launch an experiment whose results you will act on, before you see them.
Experiments require resources: budgets to collect data, engineering know-how and effort to scale treatments, and designer resources on how to design different treatments to name a few. Answering the above questions will help you determine what it will take to design and launch an experiment whose results you will act on, before you see them.
Embracing the Science of Experimentation:
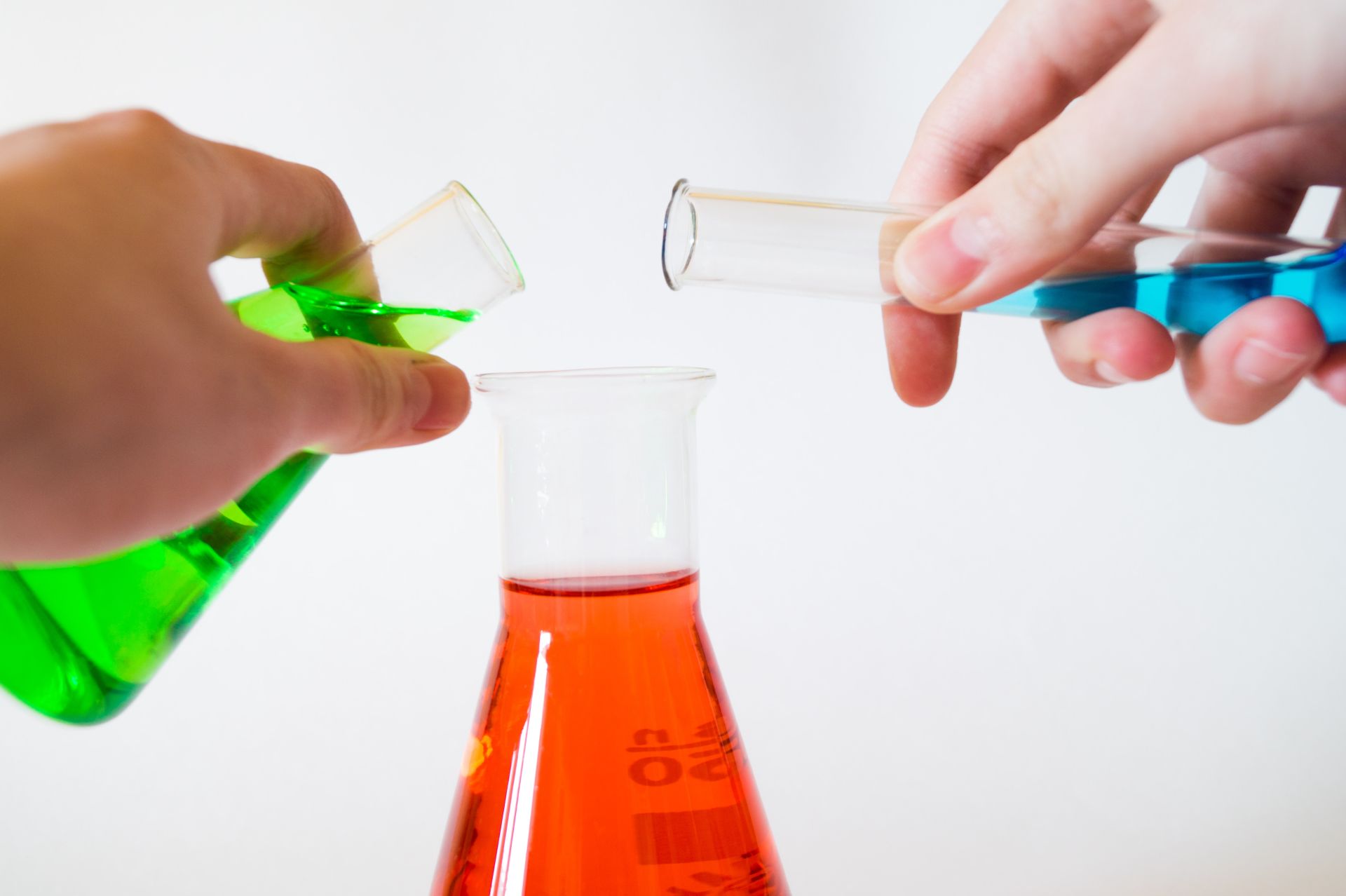
These questions are supported by experimentation science as well. They help formalize your business intuition about your problem space, and scientific knowledge about your data. For example, the risk behind the decision is formalized through knowing your tolerance for making False Positive or False Negative decisions. Dealing with the noise of your data involves sampling methods or predictive machine learning models, and the reasonable impact uses one of the core tools of experimental design, the power calculation.
Each of these questions requires its own deep dive, and our next blog post will cover the risk of making False Positive and False Negative decisions.
If you are aspiring data scientist who wants to upscale or pivot into the tech field, we have the right mentors to guide you. Check out our course Product Data Science, and the benefits you get through enrollment!
About the authors
Manish Arora
Lead Data Scientist,
Google
Lead Data Scientist,
Manisha has 10+ years’ experience in Data Science. She is the Experimentation Lead at Google Ads. Manisha is passionate about coaching aspiring Tech Professionals and has coached 300+ data scientists over the past 4 years.
Julian Hsu
Senior Economist,
Amazon
Senior Economist,
Amazon
Julian is an innovative and approachable economist with 14+ years of machine learning, experimentation, and causal ML models. With 6+ years of cross-functional collaboration, he works with product, science, and tech teams to launch productionized solutions.
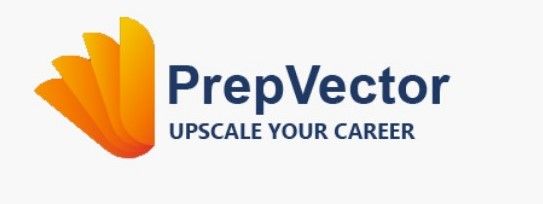
Copyright © 2022
Contact Us!
Got a question? Reach out to us and we will get back to you ASAP!
Thank you!
One more step!
Just a few details before you can download the resources.
Thank you!
Download your resource here
Download your resource here
One more step!
Just a few details before you can download the resources.
Thank you!
Download your resource here
Download your resource here
By submitting this form, you consent to abide by the Privacy Policy outlined by PrepVector.
One more step!
Just a few details before you can download the resources.
Thank you!
Download your resource here
Download your resource here
One more step!
Just a few details before you can download the resources.
Thank you!
Download your resource here
Download your resource here
One more step!
Just a few details before you can download the resources.
Thank you!
Download your resource here
Download your resource here
One more step!
Just a few details before you can download the resources.
Thank you!
Download your resource here
Download your resource here
One more step!
Just a few details before you can download the resources.
Thank you!
Download your resource here
Download your resource here
One more step!
Just a few details before you can download the resources.
Thank you!
Download your resource here
Download your resource here
One more step!
Just a few details before you can download the resources.
Thank you!
Download your resource here
Download your resource here
One more step!
Just a few details before you can download the resources.
Thank you!
Download your resource here
Download your resource here
Access has ended, sorry.
But you can reach out to us at operations@prepvector.com if you need access to the giveaway.
One more step!
Just a few details before you can download the resources.
Thank you!
Download your resource here
Download your resource here
One more step!
Just a few details before you can download the resources.
Thank you!
Download your resource here
Download your resource here
By submitting this form, you consent to abide by the Privacy Policy outlined by PrepVector.
One more step!
Just a few details before you can download the resources.
Thank you!
Download your resource here
Download your resource here
By submitting this form, you consent to abide by the Privacy Policy outlined by PrepVector.